AI and Data Science
How Can Generative AI Impact Business Intelligence?
AI and Data Science
How Can Generative AI Impact Business Intelligence?
Meet Dr Russell Hunter(Opens in a new window) – Senior Teaching Associate (Online Education and Web Technology) at the Department of Engineering, University of Cambridge and the Academic Lead for the cutting-edge Cambridge Advance Online course on Leveraging Big Data for Business Intelligence.(Opens in a new window)
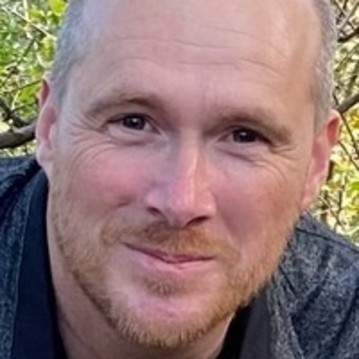
In today’s data-driven world, generative AI is revolutionising business intelligence in ways that have never been seen before. And this technology isn’t all about getting better at crunching numbers and data – it empowers businesses to make smarter, more creative decisions at the flick of a switch.
Generative AI is changing how businesses operate – going beyond just predicting outcomes to actually creating new data and ideas, imagining countless scenarios and coming up with solutions that humans might never have dreamed about.
The US management consultancy firm McKinsey predicts that generative AI will significantly impact bottom lines across all industry sectors, particularly banking, high tech and life sciences. Their latest report(Opens in a new window) estimates that generative AI’s impact on productivity could add trillions of dollars to the global economy. Across the 63 industry cases they analysed, generative AI added the equivalent of $2.6 trillion to $4.4 trillion annually. To put this into context, the United Kingdom’s entire GDP at the end of 2023 was $3.7 trillion.
So, what exactly is generative AI, and why should businesses care? In this article, we ask Dr Russell Hunter – Senior Teaching Associate at the University of Cambridge and the Academic Lead for Cambridge Advance Online’s cutting-edge Leveraging Big Data for Business Intelligence – to share his insights on how businesses can harness generative AI to transform the ways we gather business intelligence.
What is generative AI?
To appreciate the profound impact of generative AI when it comes to business intelligence, we asked Dr Hunter to explain exactly what generative AI is.
‘Generative AI refers to a subset of artificial intelligence that is capable of generating new data or content that is similar to the data it was trained on,’ he explains. ‘It uses algorithms and models to create text, images, music and other types of content that is often indistinguishable from content created by humans.’
Well-known examples of generative AI that have taken the world by storm over the past few years include:
ChatGPT(Opens in a new window) - an AI chatbot with natural language processing that allows you to have human-like conversations to complete various tasks.
DALL-E(Opens in a new window) - an AI system that generates realistic images and artwork from natural language descriptions.
Generative Adversarial Networks(Opens in a new window) - a class of machine learning framework that pits two neural networks against each other.
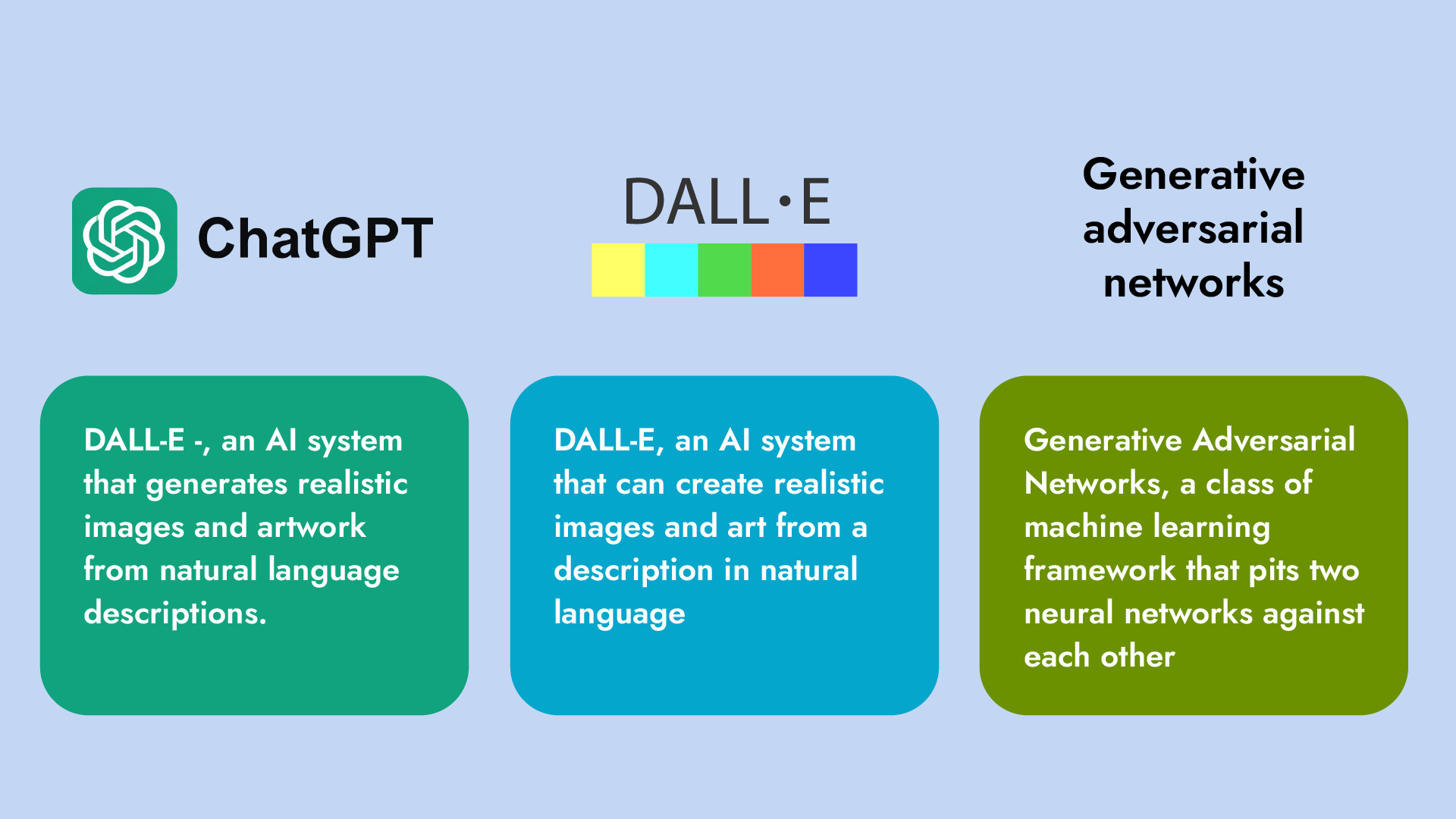
Generative AI differs from other types of AI primarily in its ability to create new content, says Dr Hunter. While traditional AI models focus on analysing and predicting based on existing data – for example, classification (that is, a model that can determine whether a customer will churn or respond to a marketing campaign), or regression (a model that can estimate how sales depend on price, advertising, seasonality among many other factors) – generative AI creates new data that expands beyond its training set.
The evolution of generative AI in business intelligence
The evolution of generative AI in business intelligence is a compelling story of growth and innovation. Initially, companies experimented with simple AI models for straightforward tasks like customer segmentation and basic sales forecasting, laying the groundwork for future advancements.
As technology advanced, particularly in AI, natural language processing and machine learning, businesses began exploring generative AI’s potential for more intricate business intelligence tasks. This included producing comprehensive market analysis reports and engaging in predictive modelling to forecast market trends and behaviours. This wasn’t just about expanding AI’s capabilities but also about enhancing how these tasks were performed, yielding deeper insights and a more nuanced understanding of data.
Today, generative AI is revolutionising business intelligence by automating content creation, enhancing data visualisation and generating insights from large datasets. This enables businesses to analyse and predict future trends, simulate scenarios and make real-time decisions. Its applications span from improving forecasting accuracy to automating the creation of reports and analyses, saving valuable time and resources.
Real-world examples of generative AI in business intelligence
The use of generative AI in the workplace has exploded over the past 12 months. Copywriting, music and advertising have all seen a massive adoption of AI in the last year too. According to McKinsey(Opens in a new window), generative AI has the potential to automate work activities that absorb 60-70% of employees’ time today.
In the retail sector, generative AI can be used to generate personalised product recommendations, create dynamic pricing strategies, optimise supply chain operations and design marketing campaigns.
As a pioneer in AI adoption, Coca-Cola has been using generative AI to reimagine everything from marketing to manufacturing and supply chain and beyond. According to a recent press release(Opens in a new window), ‘The company is currently exploring the use of generative AI-powered digital assistants...to help employees improve customer experiences, streamline operations, foster innovation, gain a competitive advantage, boost efficiency and uncover new growth opportunities.’ Last year, Coca-Cola generated an acclaimed advertising campaign called Masterpiece(Opens in a new window) using AI.
Marketing agencies are also using generative AI to create personalised marketing campaigns and content. This could be anything from emails that take recent activity and match to blog articles or content that may interest the user to social media posts designed to pique the interest of a particular user.
Utilising generative AI in business intelligence today
Generative AI is being used across various facets of business intelligence to streamline operations, forecast future trends and generate actionable insights. Dr Hunter explains them here:
Forecasting data: ‘We can leverage AI to generate new product ideas and prototypes based on market trends and consumer feedback by feeding the right data and having a deep understanding of the domain in order to make the right decisions. It helps in scenario planning by generating potential future datasets based on current trends. In analytics, it assists in identifying hidden patterns and generating insights that traditional models might miss.’
Content creation: ‘Tools like DALL-E generate complex data visualisations from textual descriptions, making data analysis more intuitive and is also useful for engaging assets, which can accelerate processes that would take up design resource.’
Personalised customer experiences: ‘Being able to drive blog post generation or social media posts at scale and with a personalised twist is something we are looking to develop now. We have investigated using Gemini and GPT.’
This goes to show how generative AI helps in automating the generation of insights from complex datasets, making it easier for analysts to identify actionable intelligence.
Read more from Dr Hunter on how businesses like Netflix and Amazon are using AI to personalise customer experiences.
Generative AI’s impact on business intelligence
As with all disruptive technologies, there are both pros and cons associated with the boom in generative AI. Let’s explore some of the positive impacts, before delving into some of the challenges and ethical considerations associated with generative AI.
Positive impacts
Increased efficiency: ‘What I have personally found useful is the speed of carrying out routine tasks,’ says Dr Hunter. ‘Where I would perhaps try to make some kind of distribution of data by hand in order to test a model out or understand a problem, I can simply ask ChatGPT, for example, by explaining it in fairly non-technical ways.’
This automation of data analysis frees up time for teams to focus on higher-level strategic activities, leading to other related benefits such as agility and enhanced decision-making.
Agility: Rapid generation and analysis of data allows quicker responses to market changes and opportunities.
Enhanced decision-making: Generative AI provides deeper, data-driven insights, enabling more informed strategic decisions.
Innovation: By identifying trends and simulating outcomes, generative AI fosters innovation in product development, marketing strategies and more. Dr Hunter emphasises, ‘With the right context it can help provide insights and potentially generate novel ideas and prototypes.’
Scalability: AI can analyse larger data sets than is humanly possible, uncovering insights that might otherwise be missed.
Personalisation: AI-driven analytics enable businesses to tailor products, services and communications to individual customer needs, enhancing engagement and loyalty.
Challenges and ethical considerations
There are also several challenges to bear in mind when using generative AI:
Data privacy: ‘The first thing that comes to mind in terms of risk is data privacy,’ says Dr Hunter. ‘When we first started working with generative AI at scale, we had to go through an extensive risk assessment process. Businesses need to make sure they implement robust data management practices to ensure data quality and privacy.’
Biase and fairness: AI systems may also inherit biases from their training data, which may lead to skewed insights. Thus, it is important to mitigate this.
Dependency risks: ‘Like with all businesses offered the chance to increase profit and reduce expenditure with a single tool, you would jump at the opportunity,’ says Dr Hunter. ‘However, the more we automate, the less human oversight we have. As we have seen in recent times, this has caused issues for some publishing companies. Also, reducing human oversight can also reduce critical thinking, which could potentially be damaging long-term.’
Integrating generative AI into business intelligence processes
For businesses that are looking to integrate generative AI into their business intelligence framework, Dr Hunter recommends several actionable strategies:
1. Define objectives clearly: Identify specific business intelligence goals and challenges where generative AI can add value.
2. Start small: Implement generative AI in smaller, manageable projects to gauge its impact and refine approaches accordingly.
‘As a business, we have started with small-scale projects to test and understand the capabilities and limitations of generative AI in something called the innovative guild,’ says Dr Hunter. ‘This is a group of people with knowledge and experience of machine learning trying to solve novel business problems.’
3. Ensure data integrity: Make sure you use high-quality, diverse data sets to train effective AI models.
4. Ethical guidelines: Develop ethical principles for AI use, focusing on transparency, accountability and fairness.
‘Having up-to-date and well-drawn out ethical guidelines is vital to mitigate risks related to bias and fairness when using generative AI,’ advises Dr Hunter.
5. Educate and train: Invest in AI machine learning courses for teams to build internal expertise in managing and optimising generative AI tools.
‘All of us need to have training opportunities which allow the group to accelerate with new ideas and solutions,’ says Dr Hunter.
Practical examples of implementation
The simplest generative AI solution that most online businesses have implemented is customer service chatbots.
‘Within my work, we use generative AI to create custom chatbots that can help meet the customers’ needs and understand customer intent, create data by understanding images or content that we can use for building a powerful vector search and generate user-facing content at scale,’ says Dr Hunter.
‘The benefits of these chatbots are irrefutable,’ he adds. ‘They can handle most routine enquiries and only escalate complex issues to humans, which really improves efficiency.’
Other ways that businesses have been implementing generative AI for business intelligence include:
Operational reports: ‘One of the most amazing things that generative AI can be used for is summarising large volumes of customer feedback into coherent reports, summarising data, highlighting key trends and sentiments and even synthesising customer feedback into actionable insights,’ says Dr Hunter. ‘This process is usually quite technical and lengthy and clearly this could help shape decisions like product strategies or marketing campaigns’
Market analysis: Generative AI can also be used to produce comprehensive market analysis reports to help stay ahead of the game.
Risk assessment: In addition to assessing the risks related to using AI, generative AI can be used to evaluate other potential business risks and develop mitigation strategies.
The transformative role of generative AI in business intelligence
Generative AI is revolutionising business intelligence, offering deeper insights, sparking innovation and streamlining business operations. As businesses navigate an increasingly data-driven world, mastering generative AI in business intelligence processes is going to be key to staying competitive and driving growth.
‘Although these things evolve very quickly, the future will likely see more sophisticated integrations of generative AI with real-time data analytics,’ predicts Dr Hunter. ‘In fact, if that can then be coupled with enhanced capabilities for automated decision-making, every business in the world would want to leverage that power.’
‘Staying updated with generative AI advancements allows businesses to maintain a competitive edge, innovate continuously and improve operational efficiency,’ he adds. ‘I remember reading an article from 2018 describing the effects of early adopters of AI versus those who did not – and the sentiment was such that those who did not use it would never catch up.’
For professionals who are eager to master the intersection between generative AI and business intelligence, Cambridge Advance Online’s cutting-edge Leveraging Big Data for Business Intelligence(Opens in a new window) course – led by Dr Hunter – is a game-changer. The course will equip leaders with the necessary skills to understand and implement AI-driven solutions effectively, giving them the knowledge and skills to navigate the future of business intelligence with confidence.
Read a Q&A with course leader Dr Hunter to discover more about Big Data and Business Intelligence.
References:
McKinsey Digital. (2023). The economic potential of generative AI: The next productivity frontier. McKinsey Digital, 14 Jun 2023. https://www.mckinsey.com/capabilities/mckinsey-digital/our-insights/the-economic-potential-of-generative-AI-the-next-productivity-frontier#introduction(Opens in a new window) accessed 2 Aug 2024.
The Coca-Cola Company. (2024). The Coca‑Cola Company and Microsoft announce five-year strategic partnership to accelerate cloud and generative AI initiatives. The Coca-Cola Company, 23 Apr 2024. https://www.coca-colacompany.com/media-center/the-coca-cola-company-and-microsoft-announce-five-year-strategic-partnership-to-accelerate-cloud-and-generative-ai-initiatives accessed 2 Aug 2024.
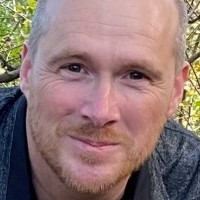